Invited Talks
Włodzisław Duch "AI: state-of-the-art and the near prospects"
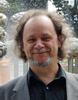
Link to CV: http://www.is.umk.pl/~duch/cv/cv.html
The future of AI is impossible to predict, but we may at least summarize the current situation and point to some promising directions for further development. Good old-fashioned symbolic AI (GOFAI) has been replaced by large neural networks in the last decade, leading to generative AI and large language models capable of associative thinking. It was insufficient to solve problems that require deeper reasoning. At the end 2024, several systems based on cognitive inspirations and search-based strategies were introduced, achieving highly significant improvements.
How far are we on the road to general high-level intelligence? In what way does computational intelligence work like biological intelligence, and how does it differ? This lecture explores the state-of-the-art in AI, delves into the challenges faced, and discusses potentially game-changing solutions that are on the horizon. We will also discuss social implications and the need for a vision of AI-empowered societies.
Guanpu Chen "Global Nash equilibrium in a class of non-convex multi-player games: Theory, algorithm and application "
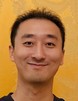
Guanpu Chen received the B.Sc. degree from the University of Science and Technology of China, China, in 2017, and the Ph.D. from the Academy of Mathematics and Systems Science, Chinese Academy of Sciences, China, in 2022. He is currently a Postdoctoral Researcher with the School of Electrical Engineering and Computer Science, KTH Royal Institute of Technology, Stockholm, Sweden. His research interests include network games, distributed optimization, and cybersecurity. Dr. Chen was the recipient of the President Award of the Chinese Academy of Sciences in 2021 and Best Paper Award at the IEEE ICCA 2024.
Many machine learning problems can be formulated as non-convex multi-player games. Due to non-convexity, it is challenging to obtain the existence condition of the global Nash equilibrium (NE) and design theoretically guaranteed algorithms. We study a class of non-convex multi-player games, where players' payoff functions consist of canonical functions and quadratic operators. We leverage conjugate properties to transform the complementary problem into a variational inequality (VI) problem using a continuous pseudo-gradient mapping. We prove the existence condition of the global NE as the solution to the VI problem satisfies a duality relation. We then design an ordinary differential equation to approach the global NE with an exponential convergence rate. For practical implementation, we derive a discretized algorithm and apply it to two scenarios: multi-player games with generalized monotonicity and multi-player potential games. Experiments on robust neural network training and sensor network localization validate our theory.
Dacheng Tao "On Championing Foundation Models"
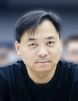
Dacheng Tao is currently a Distinguished University Professor in the College of Computing and Data Science at Singapore Nanyang Technological University. He mainly applies statistics and mathematics to artificial intelligence, and his research is detailed in one monograph and over 500 publications in prestigious journals and proceedings at leading conferences, with best paper awards, best student paper awards, and test-of-time awards. His publications have been cited over 130K times and he has an h-index 176+ in Google Scholar. He received the 2015 and 2020 Australian Eureka Prize, the 2018 IEEE ICDM Research Contributions Award, 2020 research super star by The Australian, the 2019 Diploma of The Polish Neural Network Society, and the 2021 IEEE Computer Society McCluskey Technical Achievement Award. He is a Fellow of the Australian Academy of Science, the Royal Society of NSW, the World Academy of Sciences, AAAS, ACM and IEEE.
After 80 years of development, neural networks have once again proven their value in the era of foundation models. Since the success of ChatGPT, the evolution of foundation models has been rapid, to the extent that it can almost be seen as a social movement. Developing these models requires immense human, material, and financial resources, and in a way, it represents a contest between humanity and nature. With the emergence of supermodels like GPT-4V, we find ourselves once again at a crossroads in the development of neural networks. As the scale of models continues to expand, we have witnessed many astonishing breakthroughs, even sparking discussions of the dawn of AGI (Artificial General Intelligence). However, we've also encountered significant challenges, especially in areas of trustworthiness and safety, which seem to be shrouded in uncertainty. Thus, today we must deeply reflect on both the principles and techniques of large models, and seek renewal from the pressures of competition.